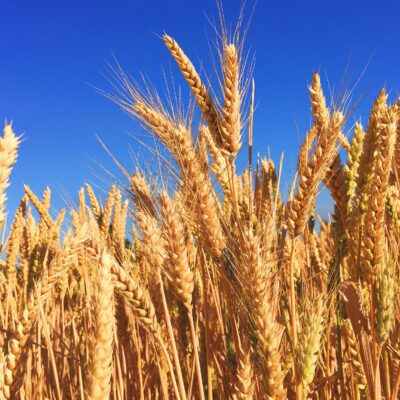
What’s up with Salmonella spp incidents: Exploration of Risks and Innovations in Food Safety
Salmonella spp. has been a common biological hazard across various outbreaks and food safety incidents over the past year. More specifically, in 2023 there has been a number of food safety incidents that were attributed to Salmonella spp.. Most notably, there were Salmonella spp. related outbreaks regarding:
- fresh diced onions in the US
- eggs in Sweden
- Cantaloupe melons in the US
- Ground beef in the US
- Flour in the US
These incidents regarding the biological hazard highlight the increasing risk of Salmonella spp. across different product categories and in different conditions. Furthermore, it showcases a growing trend for biological hazards where they are reported in food sources and conditions that we weren’t used to detect them before.
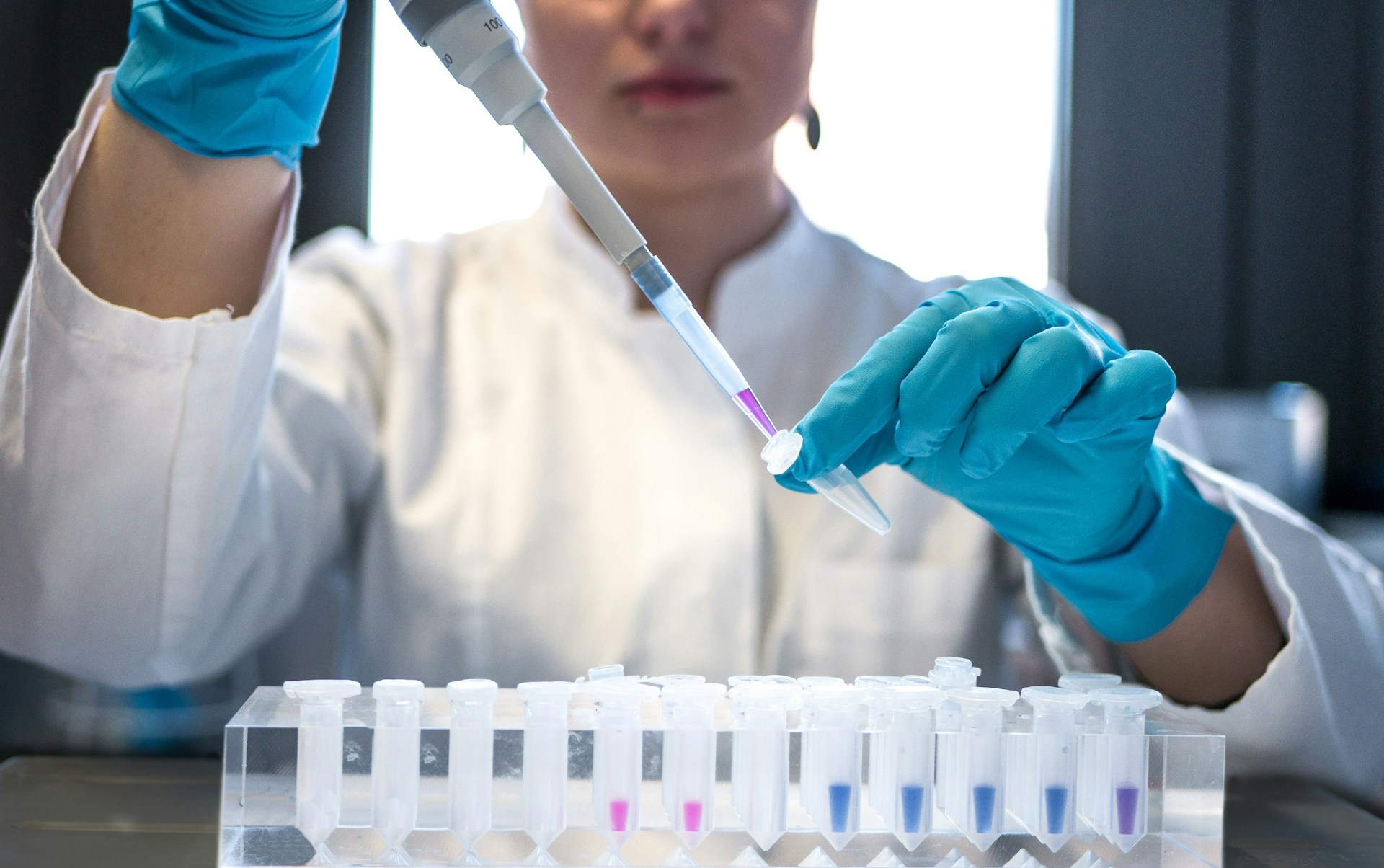
Innovation and Vigilance for a Safer Future:
Considering the persistent challenges posed by Salmonella spp., there is a growing need for innovative solutions that offer rapid, accurate, and efficient pathogen detection. As we navigate the complexities of food safety, a collective effort is essential to stay ahead of emerging threats.
Vigilance, transparent communication, and swift actions are key in safeguarding public health against the persistent and evolving risks associated with Salmonella spp. contamination. Embracing technological advancements and best practices in the food industry will play a crucial role in mitigating Salmonella spp.'s impact on global health.
Additionally, understanding Salmonella spp.'s behavior is paramount for developing robust predictive models that can revolutionize food safety practices. By leveraging technological innovations, data-driven insights, and collaborative efforts, the goal is to predict, prevent, and effectively manage Salmonella spp. contamination risks.
Predictive models play a pivotal role in understanding Salmonella spp. behavior, identifying potential contamination sources, and optimizing preventive measures. Here's a closer look at how predictive models are revolutionizing Salmonella spp. management:
1. Data-Driven Predictive Modeling: Utilizing vast datasets including factors such as temperature, pH, and historical contamination incidents, predictive models harness the power of machine learning algorithms. These models can forecast the likelihood of Salmonella spp. occurrence in specific environments, aiding in the proactive implementation of preventive strategies.
2. Environmental Monitoring: Predictive models integrate real-time environmental monitoring data, providing insights into the dynamic conditions that influence Salmonella spp. behavior. By analyzing these data streams, the models can identify trends and anomalies, allowing for swift interventions before widespread contamination occurs.
3. Supply Chain Analysis: Incorporating the entire food supply chain into predictive models enables a comprehensive assessment of potential risk points. From farm to table, these models identify critical nodes where Salmonella spp. may proliferate, allowing stakeholders to implement targeted control measures.
4. Outbreak Prediction: Analyzing historical outbreak data, predictive models can identify patterns and commonalities in Salmonella spp. occurrences. This information enables health authorities and food producers to anticipate potential outbreaks, implement preventive measures, and minimize the impact on public health.
5. Root Cause Analysis: When contamination incidents do occur, predictive models facilitate efficient root cause analyses. By tracing back the variables and conditions present during the occurrence, these models help identify the origin of the issue, enabling targeted corrective actions to prevent future incidents.
6. Integration of Genomic Data: Advancements in genomics contribute to predictive models by analyzing Salmonella spp. strains at a molecular level. This allows for a more precise understanding of strain characteristics, aiding in predicting the potential for virulence and antimicrobial resistance.
What’s the forecast for Salmonella spp. in the coming months?
As mentioned above, outbreak and incident forecasts are one way how predictive models can be utilized to revolutionize the management of Salmonella spp..One such example is the forecast of incidents trend for the number of incidents of Salmonella spp. through FOODAKAI, the AI-powered and predictive analytics food safety risk platform.
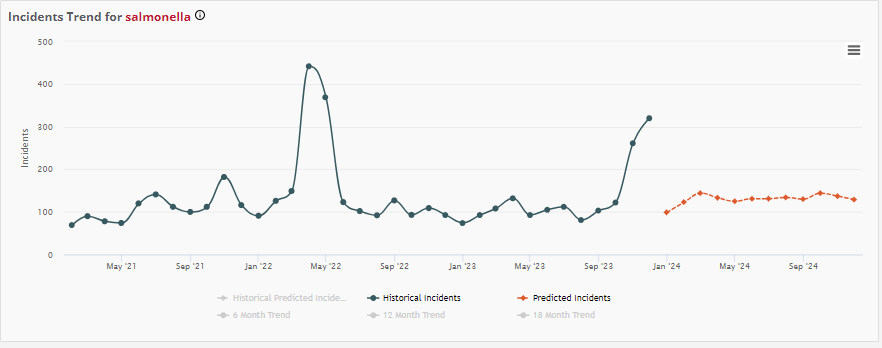
As indicated above, in the next months a slight decrease in the overall number of incidents regarding Salmonella spp. across all food categories is forecasted.
In conclusion, predictive models in Salmonella spp. management represent a paradigm shift in food safety. By harnessing data, technology, and a holistic approach to the food supply chain, these models, as evidenced with the example above, empower stakeholders to predict, prevent, and mitigate the risks associated with Salmonella spp., ultimately contributing to a safer and more resilient food ecosystem.
Want to receive helpful food safety intelligence in your inbox?
Funding for this research has been provided by the European Union’s Horizon Europe research and innovation programme EFRA (Grant Agreement Number 101093026). Funded by the European Union. Views and opinions expressed are however those of the author(s) only and do not necessarily reflect those of the European Union or European Commission-EU. Neither the European Union nor the granting authority can be held responsible for them.