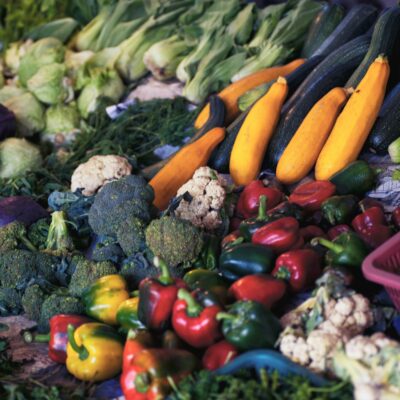
Fostering Public Health Through Data Sharing: The Crucial Role of AI in Food Safety
Food safety is an area of paramount concern for public health, as the food supply chain carries inherent risks. Contaminated food consumption has been responsible for causing widespread illness, affecting millions of individuals worldwide, with a continuous stream of reported foodborne incidents on a global scale. Consequently, the public health sector requires substantial support to effectively manage and respond to foodborne outbreaks.
In the context of food safety, the vital need for data sharing and the development of AI models to safeguard public health becomes abundantly clear. With the persistent threat of foodborne illnesses and contamination in the global food supply chain, comprehensive and timely data sharing stands as a cornerstone for effective prevention and response. Collaboration among different commodities and the sharing of data are crucial, ensuring that AI-driven solutions assume a pivotal role in enhancing food safety measures and preserving public health on a global scale.
Furthermore, this collective effort plays an essential role in advancing state-of-the-art digital infrastructure tailored for food safety. Such infrastructure not only upholds consumer safety but also instills confidence in the consistent delivery of safe products by the food industry to its consumers.
Correlation Epidemiological Studies with Food Safety Incidents
In the context of food safety, epidemiological studies can be used to identify outbreaks of foodborne illness and trace them back to their source. This helps public health officials to determine the specific foods or production processes that are responsible for the outbreak, and to take action to prevent future outbreaks from occurring.
After designing a map of outbreaks based on public health data, the next step is to analyze the correlation between these outbreaks and any announcements of food safety incidents. This can help to identify potential sources of contamination and improve food safety practices. For example, if an outbreak of foodborne illness is reported in a particular region, public health officials can check if there were any recalls of food products from that region around the same time. If a correlation is found, this may suggest that the recalled food products were the source of the outbreak.
Similarly, public health officials can compare the characteristics of the outbreaks on the map to any known food safety risks or hazards. For example, if an outbreak of E. coli is reported in a particular region, officials can investigate whether there are any nearby cattle farms that may be a potential source of contamination.
Identify Gaps in Food Safety Systems
Analyzing the correlation between outbreaks and food safety incidents can also help to identify potential gaps in the food safety system. For example, if a particular type of food product or a specific geographical region is consistently linked to outbreaks of foodborne illness, this may suggest that additional measures are needed to improve the safety of that food product. Analyzing the correlation between outbreaks and food safety incidents can be an important step in improving food safety practices, by helping policymakers and public health officials to make evidence-based decisions about food safety regulations and guidelines after evaluation of existing intervention and policies.
Identifying Correlation between Different Strains and/or Serovars of Pathogens
Through the careful examination of data acquired from public authorities and food industry, it becomes feasible to establish correlations among different strains and/or serovars of pathogens. For example, the use of antibiotics in animal agriculture is one of the primary contributors to the rise of antibiotic resistance genes. When antibiotics are routinely given to animals to promote growth and prevent disease, it creates an environment in which bacteria can develop resistance.
Genes prediction of antibiotic resistance genes based on food safety incidents, epidemiological data, and outbreaks is an important tool for public health officials and researchers in the fight against antibiotic resistance. By identifying potential sources of antibiotic resistance, appropriate treatment strategies can be developed, and public health policies can be informed to help combat this growing concern. This analysis has the potential to unveil the existence of antibiotic resistance genes within specific pathogens, while also shedding light on their geographical origins.
Predictive Modeling for the Likelihood and Severity of Foodborne Virus Outbreaks
Furthermore, predictive models for the spread of foodborne viruses across countries have become invaluable tools in ensuring public health and safety. These models can utilize a range of data sources, including historical outbreak records, epidemiological data, food safety incidents, and global travel patterns, to forecast the potential dissemination of foodborne viruses. Integrating various factors such as international trade, transportation networks, climate conditions, and population demographics, these models can provide insights into the likelihood and severity of foodborne virus outbreaks. They help identify high-risk regions, vulnerable populations, and potential routes of transmission, enabling proactive measures to be taken to prevent and mitigate the impact of outbreaks.
One of the primary benefits of these predictive models is their ability to alert health authorities and policymakers to potential emerging threats. By analyzing patterns and trends, these models can identify early warning signs of a possible outbreak and provide crucial information for implementing targeted surveillance, improving food safety regulations, and designing effective prevention strategies. Furthermore, predictive models facilitate international collaboration and information sharing between countries. By sharing data and collaborating on predictive models, countries can enhance their preparedness and response capabilities, reducing the spread of foodborne viruses and minimizing their impact on public health.
In conclusion, data sharing among commodities and the integration of innovative digital infrastructure stands as pivotal elements in the effective utilization of AI models for food safety. By enriching these models with data from diverse sources and harnessing the full potential of digital infrastructure, they become more accurate, comprehensive, and proactive. This approach not only bolsters public health protection but also ensures the continual improvement and refinement of food safety practices. The culture of collaboration and data sharing among commodities, combined with cutting-edge digital infrastructure, heralds a new era in safeguarding public health and strengthening global food safety practices.
Funding for this research has been provided by the European Union’s Horizon Europe research and innovation programme HOLiFOOD (Grant Agreement Number 101059813). Funded by the European Union. Views and opinions expressed are, however, those of the author(s) only and do not necessarily reflect those of the European Union or European Research Executive Agency. Neither the European Union nor the granting authority can be held responsible for them.