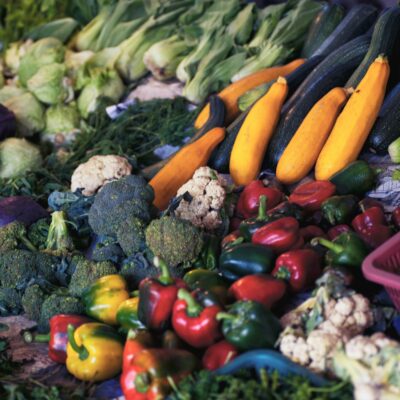
“AI is not black magic; it’s a scientific model. You train it with data, it gives back results…. It’s a constant cycle.”
Last month Agroknow’s CEO, Nikos Manoulelis was invited to an interview for the October/November Issue of the Food Quality & Safety Magazine, Farm to Fork Safety for the cover, titled “The Power of AI”.
In this latest issue that focused on AI and the power of Data sharing, Nikos Manouselis was asked by Andrea Tolu, Food Writer to contribute with his expertise and reflections on his article “Harnessing the Power of Ai: Data sharing and machine learning are transforming food safety – starting in the growing fields”.
The author’s article focused on 2023 being a revolutionary year for AI, the calls for data sharing, the readiness of the produce industry to embrace for such technologies and the possibility of new methods to lower perceived risk of data sharing (federating learning), and the applicability of AI in collecting and correlating public food safety data to extract true value. One such instance would be connecting public food safety data in order to help food & beverage companies with their food risk intelligence decision making with tools such as the generation of “predictions based on an analysis of immense volume of data”.
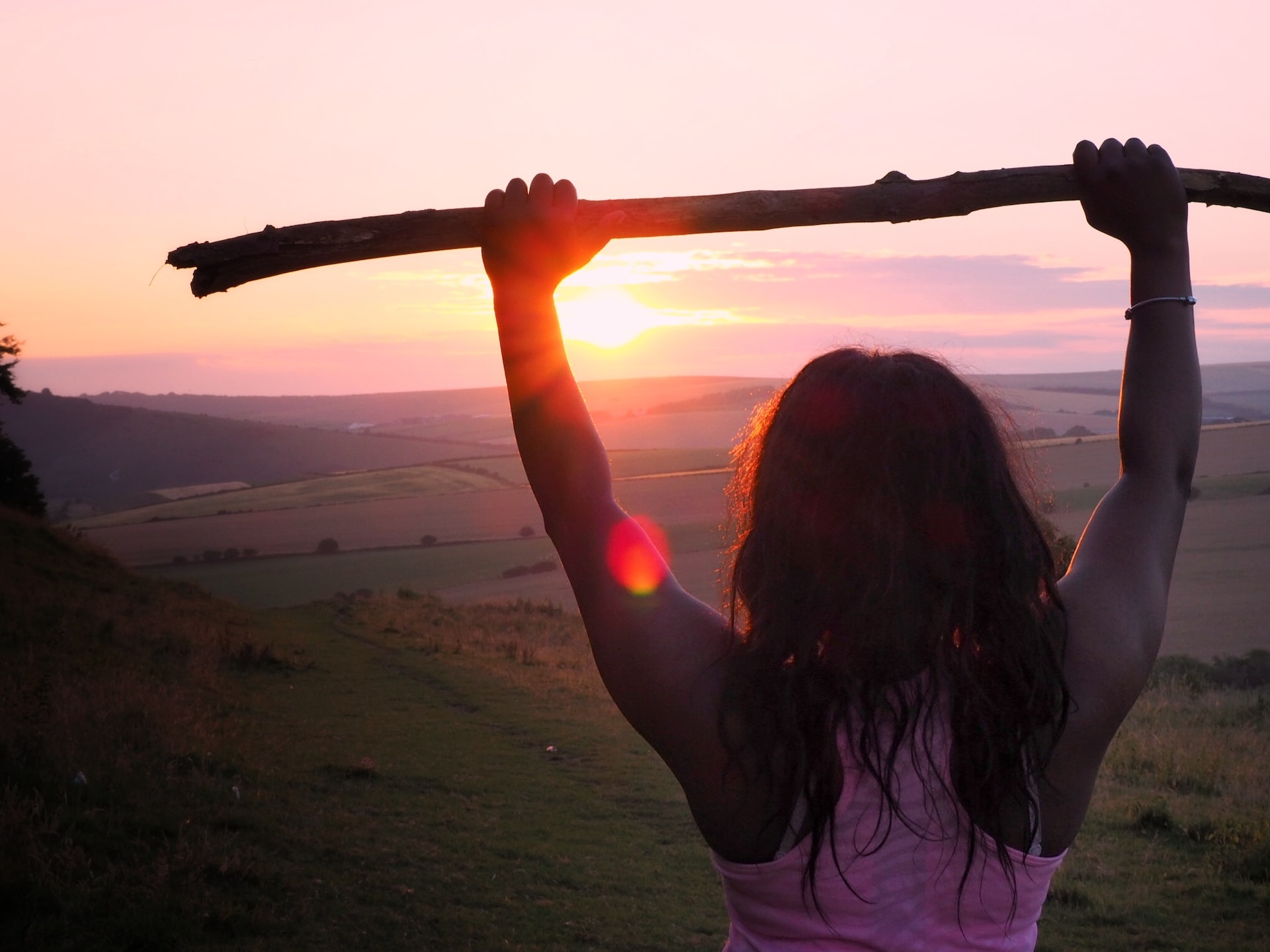
Few of the notable questions that Nikos Manouselis was invited to answer included:
How do you uncover novel data sources or useful data points that were underestimated before? What are the challenges of aggregating this type of data?
Part of our work is to discover announcements hidden in the websites of public authorities around the world and translate them into English. When the municipality of Athens inspects a food facility in the region and discovers an issue, they announce it in Greek on their website. Similarly, the FDA publishes its most important announcements in one or two pages, but there are also other pages that nobody looks at.
There may be a news article about five people who got sick from Salmonella after consuming a chicken product in Crete, and a public announcement about a recall of the same product, area, and days, where the serotype is specified. The algorithm would match them and provide a complete description of the event, assigning a reliability score.
When all this data is analyzed and harmonized with the use of AI, it gives food companies an accurate idea of the current risks in the supply chain. When their internal data, such as results of inspections, audits, and lab tests, is added, the picture is complete.
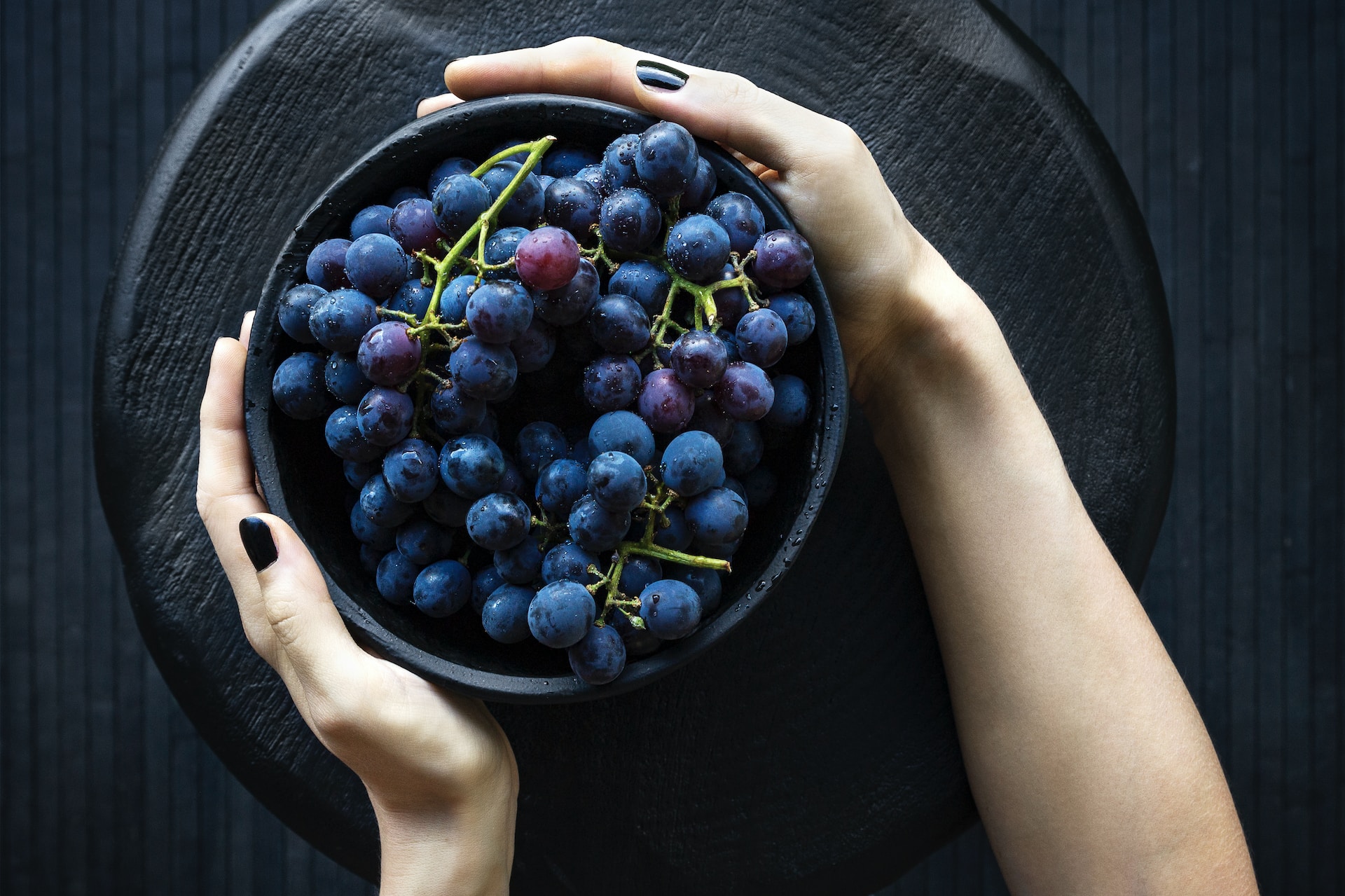
Could you please provide a couple of examples of how food businesses can use predictive analytics to minimize risk?
If there’s a spike in contaminations of ethylene oxide in sesame seeds and it’s one of my ingredients, I will know I have to test more. If one of my suppliers or other suppliers in the same area were involved in food safety or food fraud incidents, I will source from a different region.
Once you have the data, how do you use it to build an AI model that connects the dots and provides useful insights?
When we were in the middle of the ethylene oxide crisis, everyone was testing much more. At some point, our forecasting models showed that the risk was decreasing. For our clients, that was a signal that they could start testing less for ethylene oxide treatment and redirect resources to other areas.
In essence then, to help food & beverage companies to anticipate trends to better allocate testing and auditing resources, which is especially important for large food companies.
AI & Predictive Modelling Moving Forward
As food safety organizations are increasingly joining the AI movement, the calls for data sharing are growing and in a time where public authorities such as the FDA are recognizing the importance of data analytics by including it in its New Era of Smarter Food Safety Blueprint, it is of utmost importance that we aid the food safety industry to better understand the value of such technologies in enhancing food safety of their products across and wide.
Read the full article here.